What is Data Science? Data science is an interdisciplinary field that combines computer science, statistics, and domain-specific knowledge to extract useful insights from data. It makes use of a number of techniques, such as data mining, machine learning, and predictive analytics, to examine both organized and unstructured data and find trends, patterns, and connections.
Core Components of Data Science:
- Collecting data from several sources (including databases, web scraping, and sensors) and cleaning it to guarantee correctness and consistency is important because jumbled data might produce inaccurate findings.
- Using statistical and analytical methods to examine datasets in order to find trends, abnormalities, and connections that could assist guide decisions is known as data analysis and exploration.
- By using algorithms to create predictive models, machine learning and modeling enable the system to recognize patterns in data and generate well-informed predictions. In fields like fraud detection, medical diagnosis, and recommendation systems (like Netflix and Amazon), this is extensively utilized.
- Data visualization is the process of presenting data in the form of graphs, charts, and dashboards to help stakeholders who lack technical skills understand and comprehend complex information.
- Deployment and Maintenance: Models and insights from applied data science are frequently implemented in real-world settings where they continually guide or automate decision-making.
READ MORE: METAVERSE
Applications of Data Science:
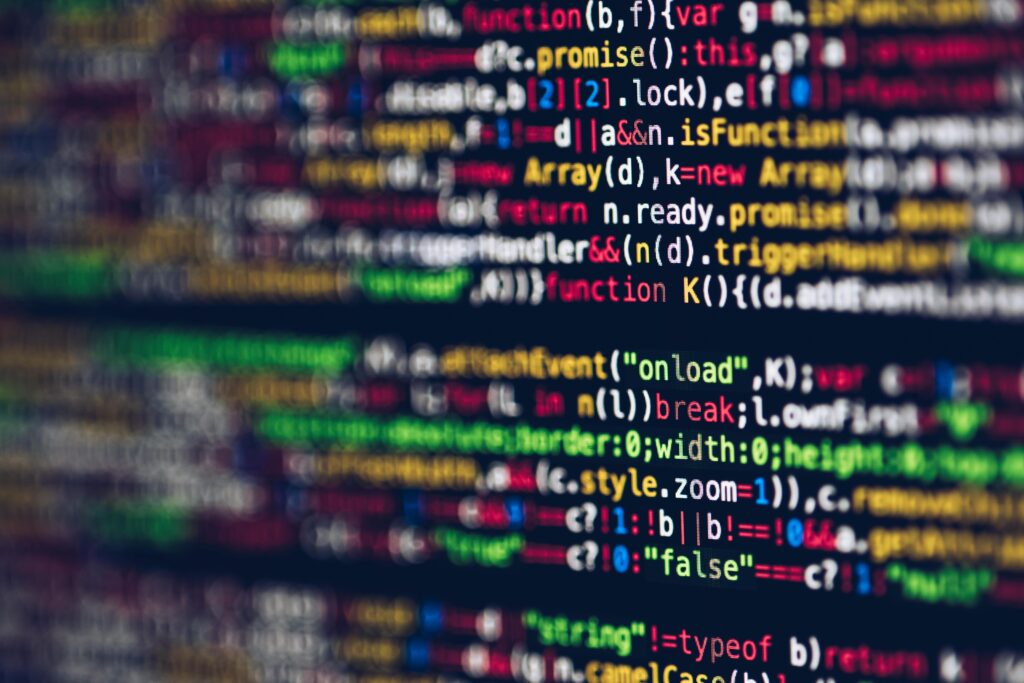
- Healthcare: Using patient data to tailor treatments, enhance diagnostics, and forecast disease outbreaks.
- Finance: Predictive analytics for stock portfolio optimization, risk management, and fraud detection.
- Marketing includes campaign optimization, consumer behavior analysis, and ad personalization.
- Improving recommendation systems, forecasting customer demand, and customizing purchasing experiences are all aspects of e-commerce.
- Social media: Content recommendations, sentiment analysis, and focused advertising.
Key Skills in Data Science:
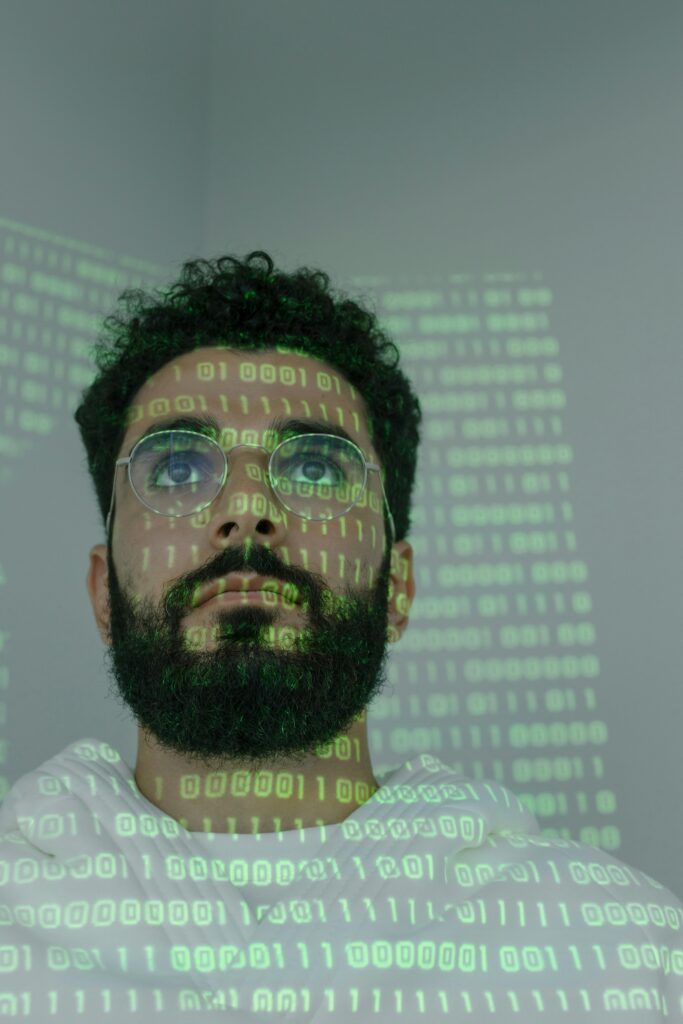
- Programming: The ability to manipulate, analyze, and create models using languages like Python, R, and SQL.
- Mathematics and Statistics: Data analysis and machine learning model development require a solid basis in linear algebra, probability, and statistics.
- Understanding supervised, unsupervised, and reinforcement learning methods is essential for machine learning.
- ETL (Extract, Transform, Load) and data wrangling: The ability to organize, clean, and transform raw data for analysis.
- Data Visualization: For visual storytelling with data, tools like Tableau, Power BI, and Python libraries (like Matplotlib and Seaborn) are essential.
- Big Data and Cloud Computing: To effectively store, process, and analyze enormous volumes of data, data science is depending more and more on big data platforms like Hadoop and Apache Spark as datasets expand at an exponential rate. Data scientists may do complicated analyses without being constrained by local hardware thanks to scalable resources offered by cloud services like AWS, Google Cloud, and Microsoft Azure.
- Analyzing and drawing conclusions from text data is the main goal of natural language processing, or NLP, a branch of data science. Text summarization, chatbots, machine translation, and sentiment analysis are a few examples of applications. By using natural language processing (NLP) approaches, businesses can better comprehend consumer opinions, improve customer service, and create automated replies that increase customer engagement.
- Deep Learning and Neural Networks: Deep learning is a branch of machine learning that analyzes large datasets using neural networks, frequently for challenging tasks like speech synthesis, picture recognition, and autonomous driving. Computer vision, speech recognition, and generative AI have all advanced as a result of deep learning models pushing the limits of artificial intelligence.
- Ethics and Data Privacy: Since data science has the ability to examine sensitive data, ethical issues are vital. Particularly when data science affects fields like criminal justice and healthcare, concerns like data privacy, algorithmic bias, and model openness are coming under more and more scrutiny. Data scientists must be on the lookout for ethical data practices and compliance because laws such as the General Data Protection Regulation (GDPR) place a strong emphasis on responsible data handling.
- Building the infrastructure that enables data to move smoothly between systems—from collection to processing and storage—is the main goal of data engineering. Data scientists can access clean, structured data thanks to the infrastructure and pipelines built by data engineers. They guarantee that data is gathered, processed, and made available for study, which makes them an essential part of the data science lifecycle.
Emerging Trends in Data Science:
- What is Data Science? Data scientists can increase efficiency and non-experts can create models thanks to AutoML (Automated Machine Learning), which makes it possible to automate some steps of the machine learning process. Tasks like feature engineering, model selection, and hyperparameter tuning are made easier by platforms like Google AutoML and H2O.ai.
- Explainable AI (XAI): Deciphering and analyzing complex models is essential as AI applications expand. Explainable AI aims to increase trust in AI systems, particularly in delicate industries like healthcare and finance, by making machine learning models more accessible and intelligible.
- Quantum Computing: Although still in its infancy, this technology has the potential to completely transform data science by resolving issues that traditional computers are unable to handle. Complex data simulations, cryptography, and optimization could all be significantly enhanced by quantum algorithms.
- Machine Learning Operations, or MLOps: MLOps, which draws inspiration from DevOps, is concerned with implementing, tracking, and preserving machine learning models in production. By standardizing machine learning processes, it seeks to guarantee that models are reliable, scalable, and simple to maintain over time.
- Augmented Analytics: Augmented analytics automates data insights through the use of AI and machine learning. This technology bridges the gap between data science and routine business operations by enabling business users to obtain insights without requiring a high level of technical expertise.
Societal and Economic Impact of Data Science:
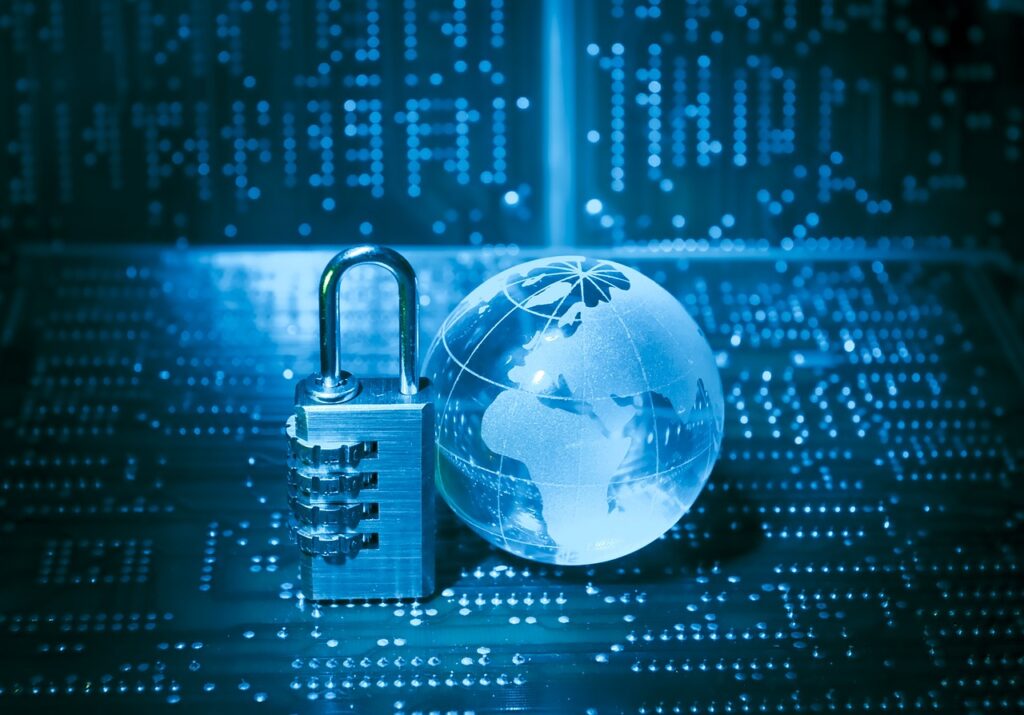
What is Data Science? Data science influences many facets of life by powering automated commercial procedures, smart cities, and tailored healthcare. It greatly increases efficiency and profitability in business by facilitating client retention, predictive maintenance, and effective supply chain management. Data science technologies help society solve social problems, distribute resources as efficiently as possible, and direct public policy. What is Data Science? Data science has a significant impact on both economic growth and public wellbeing, as evidenced by its involvement in addressing urgent issues including social inequality, global health crises, and climate change.
Key Tools and Technologies in Data Science:
- Data Processing: Python, R, SQLMachine Learning Libraries: Scikit-Learn, TensorFlow, PyTorch
- Data Visualization: Tableau, Power BI, Matplotlib, Seaborn
- Big Data Platforms: Apache Spark, Hadoop
- Cloud Services: AWS, Google Cloud, Azure
- Data Cleaning and Transformation: Pandas, Apache Beam, DataWrangler
Future of Data Science:
What is Data Science? Data science has a bright future since it will continue to spur breakthroughs in many sectors of the economy. Automation breakthroughs like AutoML and no-code platforms will enable even non-experts to use data, increasing the accessibility and adaptability of data science. Data-driven decision-making will become instantaneous as real-time data and edge computing integration grow, improving domains like financial trading and autonomous systems. Transparency and equity in AI are examples of ethical issues that are becoming more and more important, particularly as data privacy regulations change around the world. The ultimate development of quantum computing may further reshape data science by resolving issues that present computers are unable to handle, creating opportunities in intricate simulations and optimization issues.
Conclusion:
In summary, What is Data Science? the subject of data science is revolutionary, using data to spur innovation across industries, enhance workflows, and generate insights. Data science’s basis in statistics, machine learning, and computational methods enables us to interpret large datasets and use this understanding in real-world applications, such as improving corporate operations or solving global issues. As cloud storage, AI, and quantum computing develop, data science’s potential will grow, necessitating ongoing attention to data protection, ethics, and transparency. What is Data Science?After all, data science has the potential to have a hugely positive influence on global economic growth and quality of life in addition to enabling society and organizations to make well-informed decisions.
Helpfull
Pingback: Air Quality Index Trends | Are We Breathing Easier or Harder? | 2024 - ScienceSpark